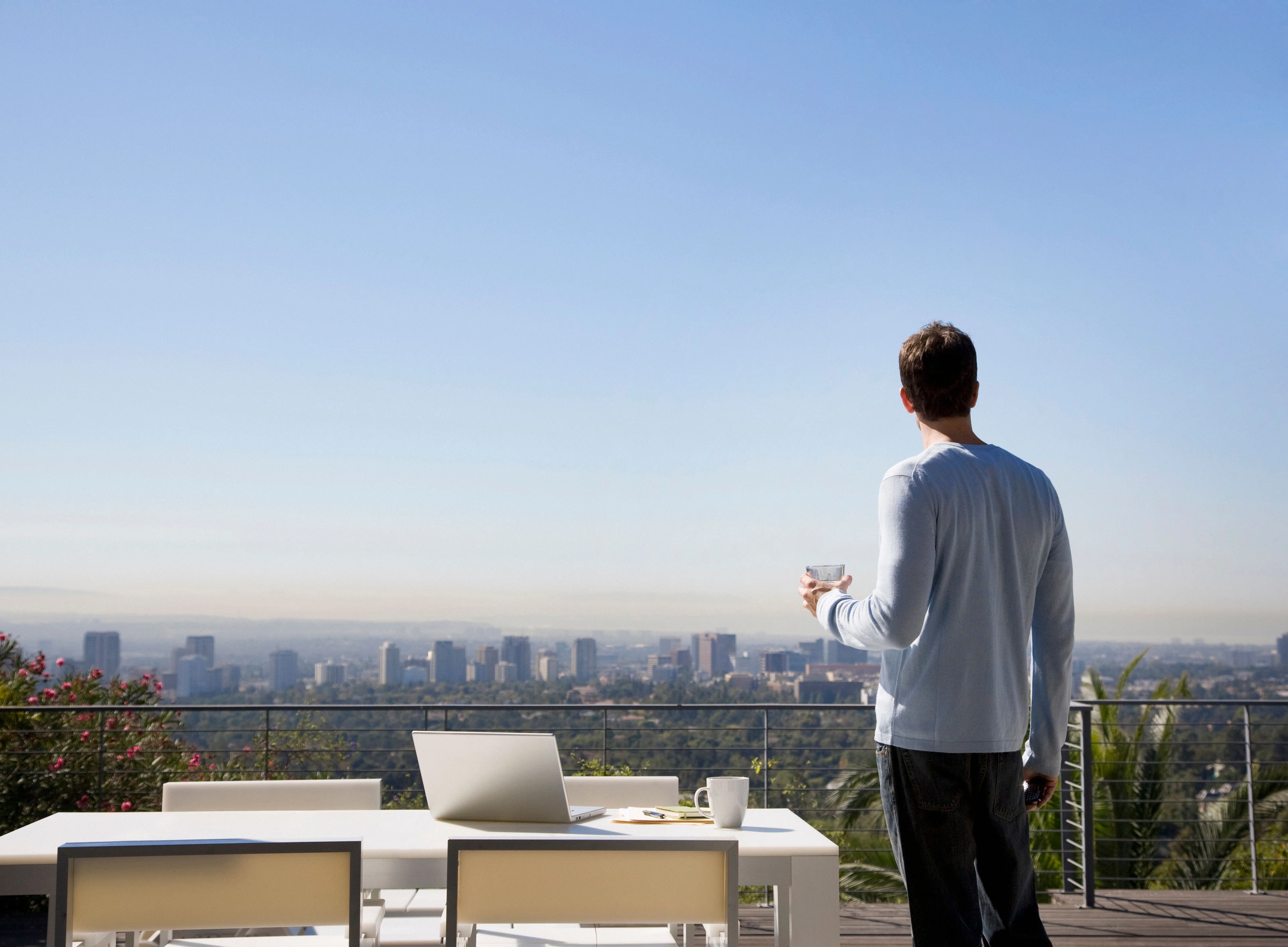
We Make AI Work for You
At Phoenix Technologies AG, we specialize in high-automation AI and Cloud Clusters, delivering scalable, sovereign, and Swiss-certified confidential computing solutions. We are redefining the future of AI infrastructure and Cloud Computing with a strong emphasis on security, scalability, and sustainability.
A trusted catalist for business transformation
As a pioneer in Sovereign AI Cloud Solutions, we empower businesses to independently create, deploy, and scale AI-driven, agentic solutions without the need for IT teams, leveraging our powerful SDK for AI agent development and customization. Our vision extends beyond infrastructure—we are actively building an ecosystem of trusted partners, enabling seamless integration, innovation, and collaboration across industries.
With full control over their data and operations, organizations can confidently embrace AI to drive bold digital transformation while maintaining sovereignty, security, and compliance. At Phoenix Technologies AG, we don’t just build AI infrastructure—we create the foundations for the future of autonomous, intelligent enterprise solutions.
Our Mission
At Phoenix Technologies AG, we build high-automation AI and cloud clusters, delivering scalable, sovereign, and Swiss-certified confidential computing solutions.
Full Service Fully Certified
Turnkey computing suite with CPU, bare-metal GPU, storage, networking, databases, HSMs, SSO, secret management, Kubernetes, VMs, and OpenShift AI.
Multi-Cloud, Multi-Architecture
Pre-configured for fast deployment across multi-architecture, multi-cloud environments, reducing setup time and complexity at no extra cost.
No Compromise
Optimize costs with automation, scalability, and efficiency while avoiding overprovisioning, licensing overhead, and hidden fees.
Confidentiality
Encrypts data at rest, in transit, and during processing with hardware security, ensuring zero unauthorized access and secure credential management.
Phoenix Technologies Partners
The Swiss Way
At Phoenix Technologies, we guarantee unmatched security and sovereignty for every solution, application, and product. By adopting latest innovations, businesses can ensure robust data protection, privacy, and control, meeting the highest standards of security and reliability.
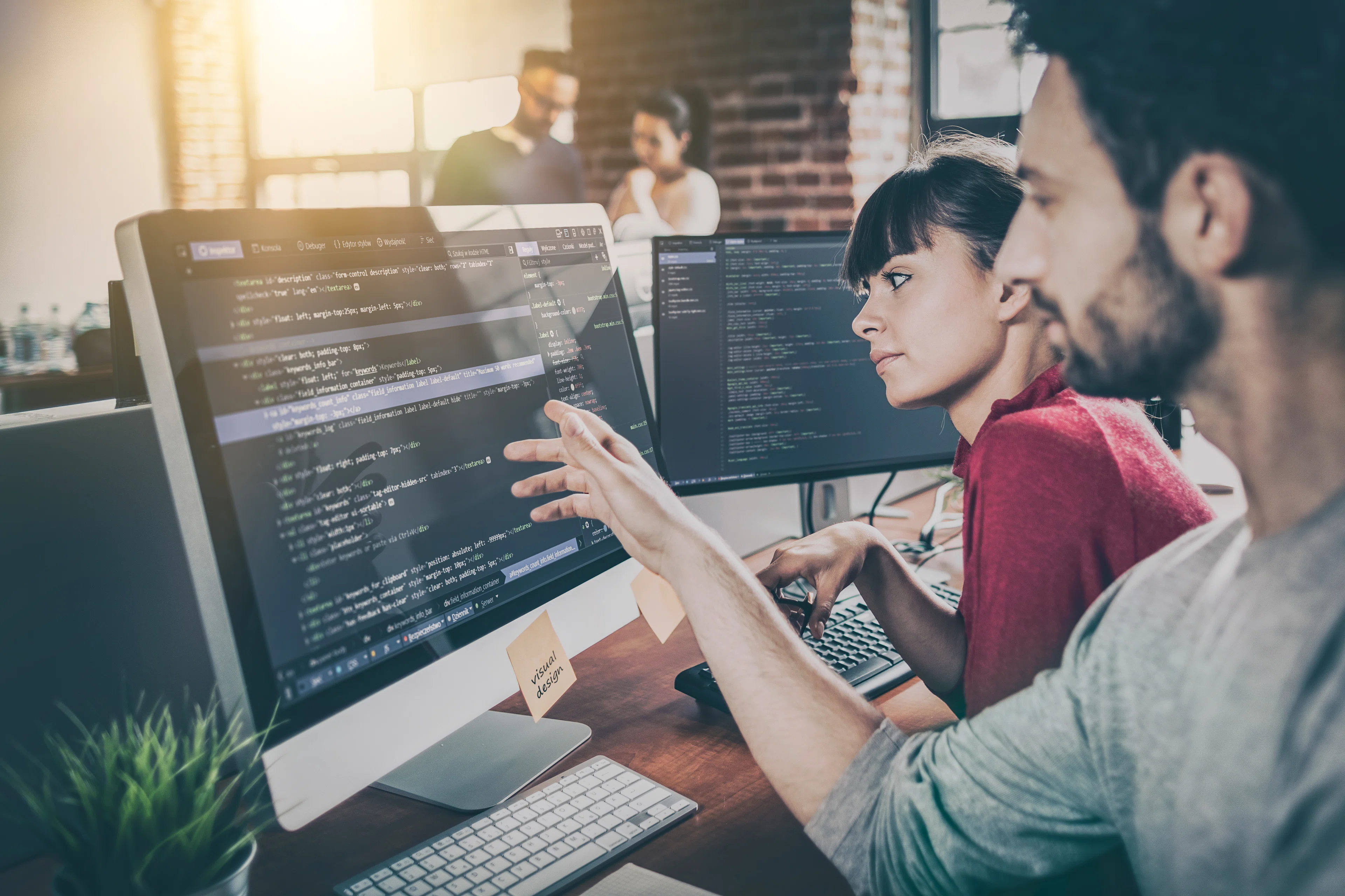